Recently, Professor Zhangsheng Yu, the founder of Intelligent Medicine Original Medical Technology (Shanghai), led his team to collaborate with an international pharmaceutical company. Their research, titled “Deep learning for oncologic treatment outcomes and endpoints evaluation from CT scans in liver cancer”, was published online in the Nature Index journal “npj Precision Oncology”. This study developed a deep learning-based tool for tumor progression evaluation, capable of automatically assessing tumor burden and identifying new lesions in longitudinal 3D CT images. The tool provides independent, stable, and objective judgments on disease progression for oncology clinical trials. Doctoral students Xia Yujia and Zhou Jie from Shanghai Jiao Tong University School of Life Sciences and Biotechnology are the co-first authors of the paper, while Professor Zhangsheng Yu, Dr. Zhao Shuai from the Affiliated Xinhua Hospital of Shanghai Jiao Tong University School of Medicine, and Dr. Zhang Jin from PiHealth are the co-corresponding authors.
Objective response rate (ORR) and progression-free survival (PFS) are commonly used endpoints in phase II/III clinical trials of anti-tumor drugs. The accuracy of these indicators depends on the precise evaluation of treatment outcomes. The standardized evaluation method RECIST v1.1 (Response Evaluation Criteria in Solid Tumors) is currently the guideline for assessing tumor response to therapy. It evaluates the tumor response based on changes in tumor burden through regular imaging follow-ups during treatment, comparing follow-up images with baseline images to determine disease remission, stability, or progression.
In clinical practice, radiologists typically identify lesions, measure tumor burden, and detect new lesions in 3D CT image sequences to monitor treatment responses. This process is extremely time-consuming and labor-intensive. Additionally, subjective interpretation can lead to discrepancies between radiologists. Studies have shown that the inconsistency rate between two readers can reach 23% to 46%. Inaccurate evaluations may result in incorrect medical decisions, affecting treatment outcomes and shortening patient survival. Furthermore, imprecise evaluation of drug treatment endpoints could prevent effective drugs from being approved or allow ineffective drugs to enter the market. Enhancing the standardization and consistency of imaging evaluations is a critical issue that needs to be addressed.
This study aimed to develop an automated tumor progression evaluation model based on deep learning. The model translates clinical radiologists’ assessment workflows into deep learning processes. It first performs precise tumor segmentation from 3D CT images, then calculates overall tumor burden changes based on baseline and follow-up images, and identifies new lesions, ultimately generating tumor progression evaluation results. Using a multitask learning framework, the model optimizes both the tumor segmentation task and the progression classification task simultaneously, integrating medical prior knowledge and correlations between longitudinal images to improve the accuracy of tumor progression evaluation in liver cancer compared to existing models.
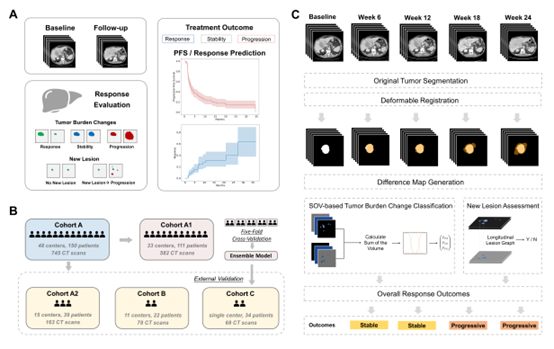
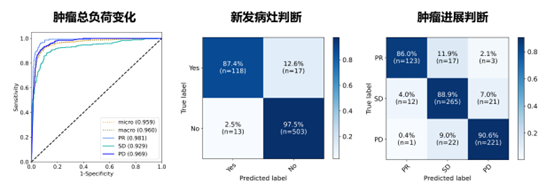
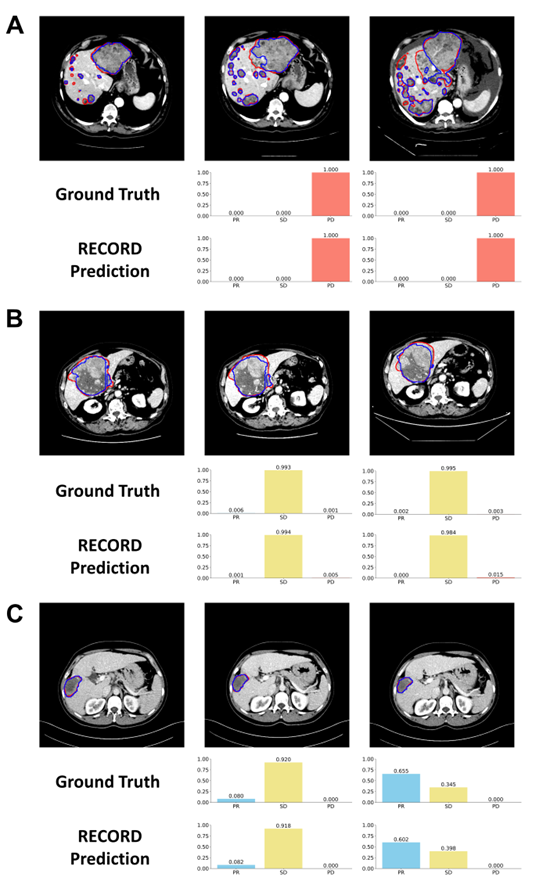
The constructed model underwent five-fold cross-validation within an international multicenter clinical trial cohort, followed by evaluation on three heterogeneous independent external validation datasets. These validation sets included primary hepatocellular carcinoma (HCC), metastatic liver cancer, and mixed immunotherapy-related liver cancer scenarios from international clinical trials and real-world practice. The model demonstrated outstanding performance across three aspects: it achieved accuracy rates of 0.927, 0.982, and 0.882 in baseline-follow-up image analysis across the three external validation cohorts, significantly outperforming existing liver tumor models in progression evaluation. For patient outcome endpoints, such as progression-free survival and drug response time, the consistency between the model’s predicted and actual outcomes reached 0.958. Additionally, the model’s disease progression assessments showed a stronger correlation with overall survival compared to manual evaluations. These results highlight the model’s potential to support independent imaging review in clinical trials, reducing subjective biases in radiologist assessments and enhancing consistency in treatment outcome evaluations across medical centers.