Recently, the team of Professor Zhangsheng Yu, the founder of the company, and their collaborators published a research paper titled “Harnessing TME depicted by histological images to improve cancer prognosis through a deep learning system” in the Cell sub-journal “Cell Reports Medicine.” This study developed a deep learning system that can predict tumor microenvironment (TME) information through histopathological images for cancer patients without spatial transcriptomics data, thereby achieving accurate cancer prognosis. This significantly expands the use of spatial gene expression information in large public biomedical pathology image databases.
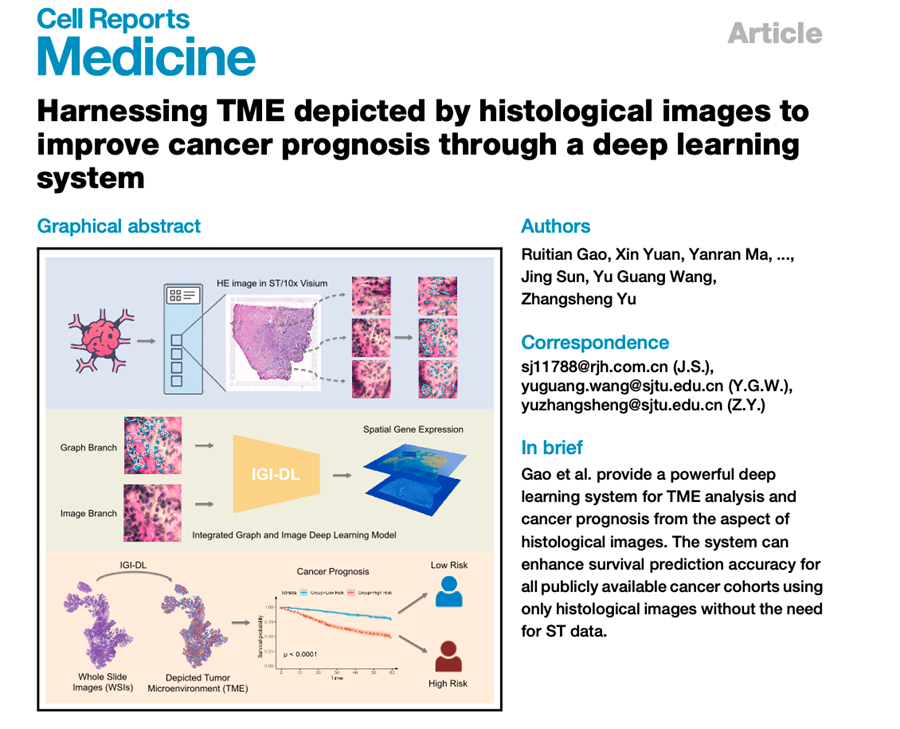
Predicting the prognosis of cancer patients has always been a significant challenge in clinical practice. The tumor microenvironment (TME) is crucial for the initiation, progression, and metastasis of solid tumors. Increasing research has revealed the correlation between the TME and cancer prognosis and treatment options. Spatial transcriptomics technology can characterize the TME from the perspective of spatial gene expression, distinguishing different prognostic subgroups of cancer patients. However, the high cost and long experimental cycles of spatial transcriptomics hinder its application in large-scale cancer patient cohorts for survival prediction. Histopathological images, which are easily accessible in clinical settings, provide rich information on tumor morphology. Developing an artificial intelligence model that can predict spatial gene expression levels at the molecular level based on these images to characterize the TME could lead to more accurate cancer prognosis.
This study aimed to develop a deep learning system that uses histopathological images to predict high-dimensional spatial gene expression levels in corresponding regions, overcoming the limitations of high costs and limited sample sizes associated with spatial transcriptomics data. This system can characterize the TME in large cancer cohorts with only pathological image data and no spatial transcriptomics data, thereby improving the accuracy of cancer patient prognosis. The deep learning system consists of two parts: the first part is a spatial transcriptomics expression level prediction model (IGI-DL) based on convolutional neural networks (CNNs) and graph neural networks (GNNs), and the second part is a cancer survival prognosis prediction based on spatial gene expression-derived TME information.
The IGI-DL model leverages the strengths of CNNs and GNNs, fully utilizing pixel intensity and structural features in histopathological images to achieve more accurate predictions of spatial gene expression levels. The model performed excellently in colorectal cancer, breast cancer, and skin squamous cell carcinoma, showing an average correlation coefficient improvement of 0.171 compared to five existing methods.
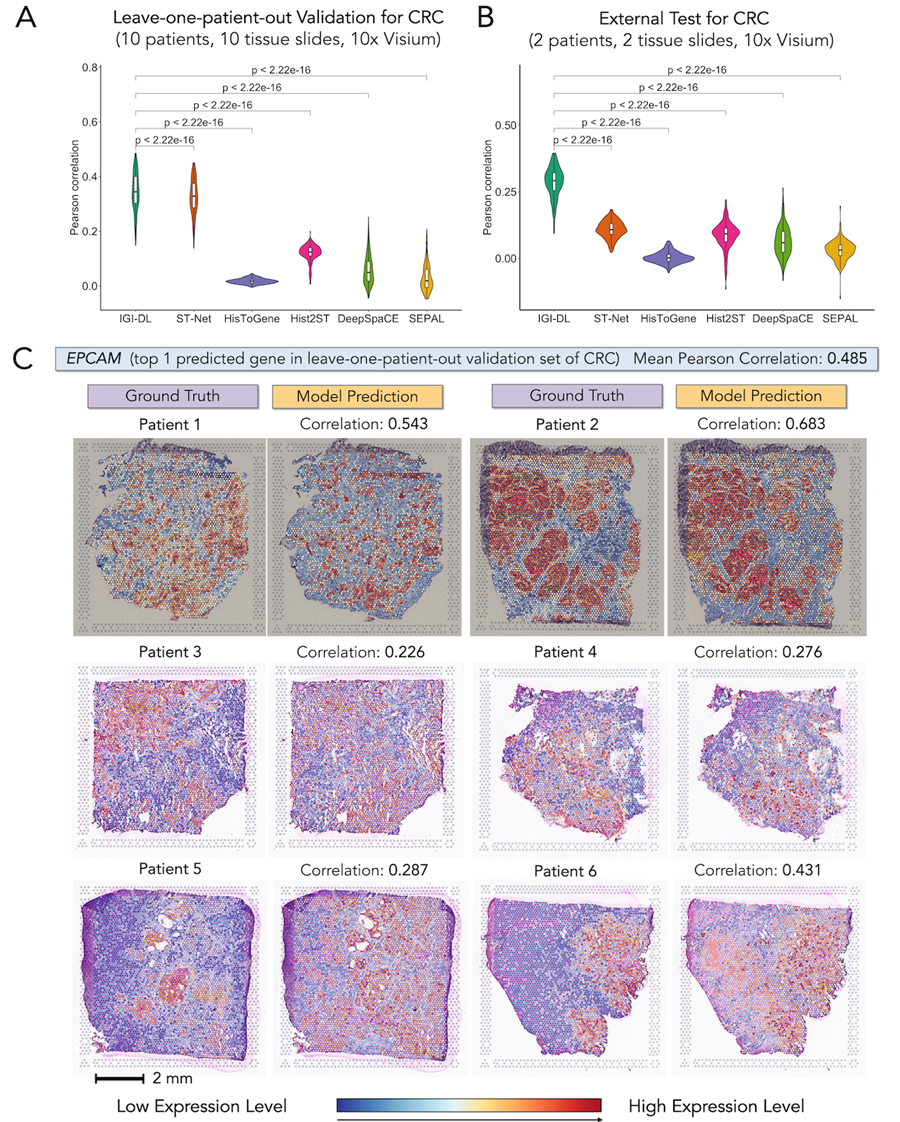
Furthermore, the IGI-DL model was applied to infer spatial gene expression from histopathological images, constructing a Super-patch graph for cancer patient survival prognosis. The study results indicate that using the spatial gene expression predicted by IGI-DL as node features in the Super-patch graph improves the performance of the survival prognosis model in the TCGA dataset for breast cancer and colorectal cancer cohorts. The five-fold cross-validation C-index was 0.747 and 0.725, respectively, outperforming other survival prognosis models. This survival prognosis model also maintained its accuracy advantage for early-stage patients (Stages I and II), with the predicted risk scores serving as independent prognostic indicators for patients at all stages and early-stage patients.
In an external test set MCO-CRC, which includes data from over a thousand patients, the survival prognosis model maintained its stable advantage, demonstrating generalizability.
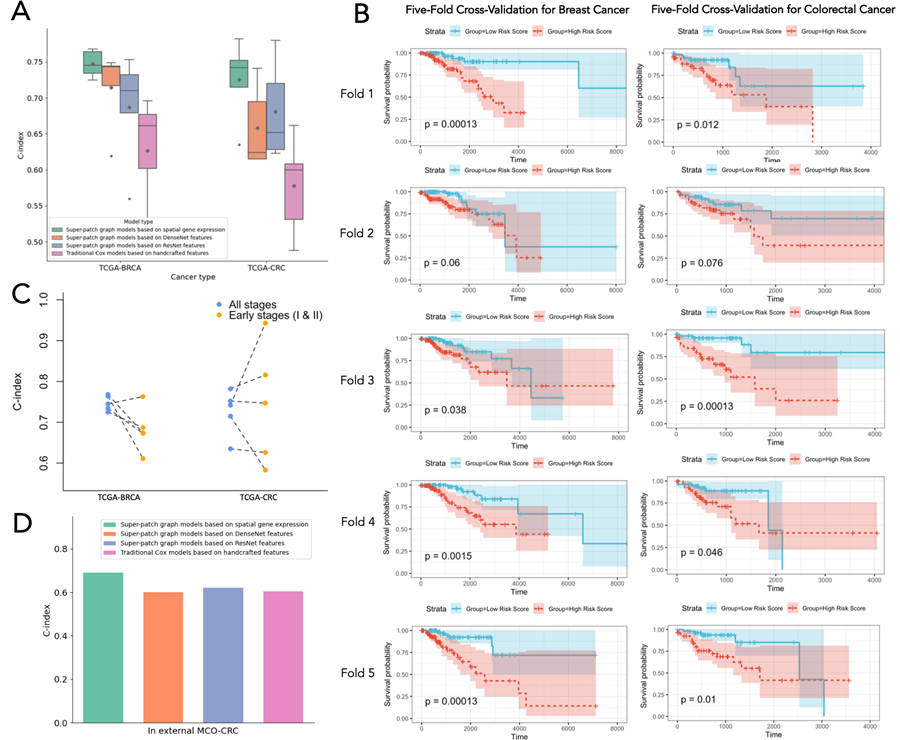
This study was supported by the National Natural Science Foundation of China, the Shanghai Science and Technology Committee Fund, and the Shanghai Jiao Tong University “Interdisciplinary Research Fund.” We also thank the Supercomputing Platform provided by the Network Information Center of Shanghai Jiao Tong University.